Predicting Internal and External Training Load with AI
- Savvas Hadjipantelis
- 2 days ago
- 5 min read
Introduction: What are Internal and External Loads?
Internal and external training loads are two key pillars of athlete monitoring. Internal training load includes physiological (e.g. heart rate, blood lactate, oxygen consumption) and psychological (RPE - ratings of perceived exertion, stress, well-being) markers, collected by sensors and questionnaires. External training load includes variables collected by electronic performance and tracking systems (velocity, acceleration, deceleration, average speed, top speed) as well as numerous other variables, such as power output and weight lifted (1). The ratio of internal and external workload variables has been demonstrated to be important as a predictor of injury.
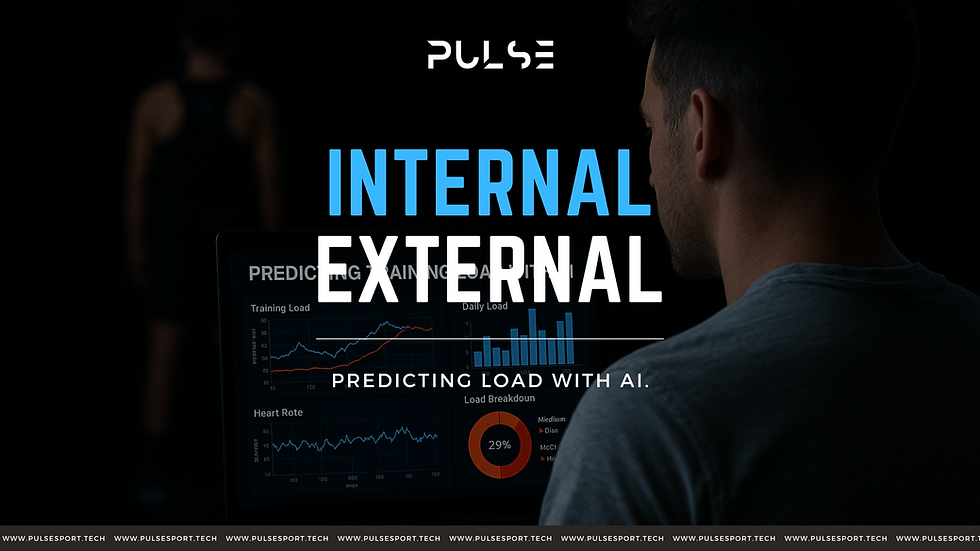
As modern sports continue to embrace data-driven approaches, Artificial Intelligence (AI) is emerging as a transformative tool in athlete monitoring and performance management. Unlike traditional methods that rely heavily on isolated metrics and coach intuition, AI can analyze vast, multidimensional datasets—including physical performance, wellness, and psychological indicators—to uncover patterns and make predictive assessments (1). Machine learning models have already started being applied in elite football environments to show any injury risk by integrating both internal and external loads (1).
What is Artificial Intelligence in Sports Monitoring?

AI and its subset Machine Learning (ML) refer to the computational techniques that allow systems to learn from data, make predictions and identify patterns with very little human intervention (2). Artificial Intelligence and Machine Learning in sports, enables practitioners to move beyond descriptive statistics towards predictive modelling and informed decision making. For example, in the study by Rojas-Valverde (2020) machine learning algorithms were used to combine internal training loads with external metrics to predict non-contact injuries in elite football players. By combining these many data streams, the models were able to detect subtle, non-linear relationships that would have been practically difficult to find with conventional techniques (2). What this does is demonstrate what AI/ML can do in automating insights, personalising training recommendations and anticipating performance or health outcomes before issues arise, making it a true game-changer in athlete monitoring!
Predicting Internal Load From External Data
Artificial intelligence can uncover connections between an athlete’s movement patterns and their physiological responses, like heart rate, without requiring direct measurement (5). By analyzing external load e.g. accelerations, speed distance, and total running volume, AI can now predict how intensely an athlete’s cardiovascular system works (5).
This approach was successfully demonstrated in a study by Gualtieri, where ML was used to develop a Fitness Index that estimated heart rate responses during training sessions. The model trained on a GPS and heart rate data, which allowed the practitioners to assess aerobic fitness without traditional testing protocols, offering a fast and scalable solution for athlete monitoring in football (5).
Combining Internal and External Load for Injury Prevention
Injury prevention in elite sport often hinges on the ability to detect overload of training before it results in any harm for the athletes. While external data offers insights into physical demands, it only tells one part of the story for athletes’ health conditions (2). Internal data such as RPE and wellness scores reflect how the body is coping with those demands. By combining both sources, AI can identify relationships and early warning signs that coaches might miss through traditional monitoring (2).
A study by Rojas-Valverde used this approach where ML models were trained on both GPS (external data) and subjective internal data to predict non contact injuries in football players. The results of the study showed that the model significantly outperformed those based on just one data type, proving how AI can uncover patterns and support a more proactive load management strategy (2).
Forecasting Wellness & Readiness with AI
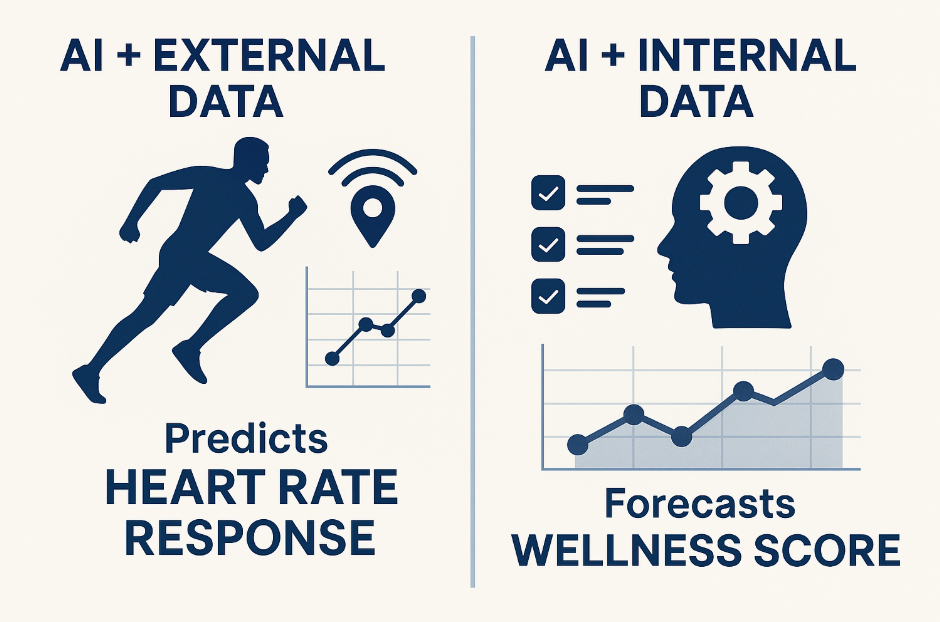
Athlete wellness is not only influenced by training, but by a more holistic approach of the general life of athletes, e.g. daily fluctuations in sleep, stress, recovery, and fatigue (3). By analyzing patterns in historical training data, both internal and external, AI models can forecast wellness scores and highlight when an athlete might be at risk of “under-recovery” (3).
This predictive approach was demonstrated in Ayala’s et al study, where ML was used to anticipate day to day wellness based on prior workload patterns of football players. The model achieved high accuracy, showing how AI can support data-driven recovery decisions and help practitioners tailor training loads to promote long-term performance and health (3).
Understanding RPE Through AI
Ratings of Perceived Exertion (RPE) is one of the most common tools used to monitor internal load, but it introduces inconsistencies across athletes and training. By applying AI to external data, (total distance, accelerations etc.) coaches can predict RPE values with more accuracy (4). This approach standardizes subjective reports, making them more reliable for performance monitoring and training adjustments.
In the study of Casamichana et al. this concept was explored as models were trained to predict football players’ RPE scores, based on GPS external metrics. The findings showed that AI could effectively connect the gap between objective and subjective monitoring, allowing coaches to better interpret athlete feedback and training loads with more precision.
Future of Athlete Monitoring: Smarter Systems
Athlete monitoring is moving beyond just predicting outcomes. With AI it is possible to build adaptive and personalized systems that adjust based on each of the athletes’ needs. By combining different types of data such as training load, sleep quality, stress levels etc, machine learning can give a clearer picture of an athlete’s health and performance (1). Instead of looking at one factor at a time, artificial intelligence connects everything to show how different stressors affect readiness and injury risk (1).
The study of Rossi et al., reviewed the role of machine learning in football injury prevention. It was shown that by combining diverse datasets you get more accurate and actionable insights than by only relying on physical metrics. As AI continues to evolve, athlete management will become increasingly personalized, proactive, and precise, helping coaches intervene earlier and optimize training on an individual level (1).
Conclusion: A Smarter Way to Coach
Machine Learning and Artificial Intelligence are now reshaping how training loads are measured and managed, offering coaches completely new ways to personalise programs for their athletes. The use of AI can detect any early warnings of fatigue or injury, and manage workloads more efficiently. By analyzing both internal and external data, AI provides a more complete and real-time understanding of an athlete’s condition. Importantly, AI is not replacing the coach’s role, but enhances it. With better insights, and use of AI coaches can make faster, smarter and more informed decisions to support their athletes.
REFERENCES
Rossi, A., Perri, E., & Lovecchio, N. (2022). Machine learning for understanding and predicting injuries in football: A systematic review. Sports Medicine - Open, 8(1), 26. https://doi.org/10.1186/s40798-022-00465-4
Rojas-Valverde, D., Gómez-Carmona, C. D., Gutiérrez-Vargas, R., Pino-Ortega, J., & Timón, R. (2020). Combining internal and external training load in soccer: Comparisons of machine learning models to predict non-contact injuries. Applied Sciences, 10(15), 5261. https://doi.org/10.3390/app10155261
Ayala, F., Robles-Palazón, F. J., & Vera-García, F. J. (2022). Wellness forecasting by external and internal workloads in elite soccer players: A machine learning approach. Frontiers in Physiology, 13, 896928. https://doi.org/10.3389/fphys.2022.896928
Casamichana, D., Castellano, J., Calleja-González, J., San Román, J., & Castagna, C. (2013). Relationship between indicators of training load in soccer players. Journal of Strength and Conditioning Research, 27(2), 369–374. https://doi.org/10.1519/JSC.0b013e3182548af1
Gualtieri, A., Rampinini, E., Sassi, R., & Beato, M. (2021). Predicting soccer players’ fitness status through a machine-learning approach using GPS and heart rate data. Global Performance Insights. Retrieved from https://www.globalperformanceinsights.com/post/new-publication-predicting-soccer-players-fitness-status-through-a-machine-learning-approach
An insightful and forward-thinking read! The real strength lies in how machine learning can detect injury risks and optimize performance through personalized, data-driven insights. An exciting glimpse into the future of smarter coaching and athlete care.
Really interesting read
This is exactly where I see the future of sports science heading. As a Sports Scientist I often find it difficult to analyze all the internal and external load data we collect, there’s simply too much of it to make sense of manually in a meaningful timeframe.
If AI offers a way to actually use that data at scale and generate insights we can act on, thats amazing. I’m not using that myself, but this post has definitely inspired me to explore. Would love to see more practical case studies or tools to get started